In This Story
An Interview with Dr. Farrokh Alemi
Faculty proposes co-teaching course aided by artificial intelligence.

Dr. Farrokh Alemi is a professor in the Department of Health Administration and Policy at the George Mason College of Public Health. Dr. Alemi’s research expertise includes the use of data mining, natural language processing, and artificial intelligence in health services research.
Could faculty members really replace themselves with artificial intelligence?
I am already working on using ChatGPT and artificial intelligence (AI) in my courses. For example, HAP 823 spring session uses the public version of ChatGPT to explain concepts to students. In fact, I’ve also proposed to “replace” myself with AI in HAP 725 Statistical Process Control. Of course, AI could not really completely replace me for student interaction, grading exams, discussion groups, etc., but there are many steps I could take to automate this course fully. Here is an example of how ChatGPT can help: instead of me teaching how to code in a control chart in python, the public version of ChatGPT can draft the code and show students how it is done. It is my goal to make HAP 725 taught entirely by AI and for me to have “a human-in-the-loop function,” backing up the AI when it fails to deliver. What better way to teach about the potential of artificial intelligence?
What does it mean to have a human-in-the-loop function?
Human-in-the-loop function means that the faculty will supervise the messages to the students, prior to the machine sending it. Students can also at any time ask for a meeting with the faculty.
If we can automate HAP 725, why would we need faculty in the classroom at all? What value does faculty provide if AI can do it for us?
Faculty still decide the content of the course and still remain as the person to respond to students. Automation allows one faculty to address a larger number of students. The role of the faculty is still critically important to teaching critical thinking skills and helping students develop skills in application and synthesis, especially innovative applications. This challenges faculty in higher education to move beyond learning mastery—a function AI may be able to support. Innovation and critical thinking...that is the domain of faculty and their teaching strategies and engagement with students.
Would ChatGPT be helpful in your current AI-powered decision aid for selecting antidepressants?
We are also working on using ChatGPT in our research. For example, we are working to improve feedback to people who use our decision aid on antidepressants (http://MeAgainMeds.com). Today, when ChatGPT answers a question on antidepressants, it gives very general advice. We need to make it very specific to be useful. Google Brain has made ChatGPT more specific by training it further. The specific version of Google Brain can pass the U.S. Medical Licensing Exam. Thus, it has more knowledge and is more specific to the exam. When it comes to antidepressant selection, none of the current versions of ChatGPT are sufficiently detailed to address the needs of patients. The system we are planning will be trained to do so.
What would it take to make ChatGPT more specific to serve your research purposes?
Unfortunately, the use of ChatGPT in our situation relies on their private engine, which is expensive. We expect simply training ChatGPT to understand formulas for diagnosis of COIVD will cost us a minimum of $5,000. It could cost significantly more, upward of $50,000. The problem is that we need to generate all combinations of variables in the model, so a simple regression model with ten variables leads to 2^10 possible text-based scenarios; a model with 20 variables leads to 2^20 combinations, and so on.
We can use LASSO regression to reduce the number of variables, but still, there are a lot of training combinations necessary. Of course, this is expected as formulas are a far more compact form of information than natural language. Nevertheless, natural language is how we talk to each other and how clinicians and patients talk, so the transition must happen.
In exploring the API of ChatGPT, we found that we can use statistical models as a component of ChatGPT. In our work, we use network models that are comprised of chain of regression equations. We are exploring how this can be built into ChatGPT.
How might you work around some of the costs associated with more specific symptoms using ChatGPT?
There are two ways to reduce the number of scenarios needed to train ChatGPT. One is to take advantage of independence structure among the variables. Our models of data provide information on independence of certain variables. For example, in COVID, we know that some symptoms' contributions to the diagnosis of COVID are independent of other symptoms. Anytime you have independence, then you can reduce the combinations of symptoms needed.
The second method is to use regression equations directly within ChatGPT. The application provides a method for combining regression equations to ChatGPT inferences. We need to combine a chain of regressions, which is a bit more complex, but it seems plausible that this can be done in the private application for ChatGPT.
How can ChatGPT help other efforts to organize natural conversations with patients?
ChatGPT can help efforts to understand patients’ open comments by providing alternative ways of saying the same words/phrases. For example, we can use the public version of ChatGPT to learn words that can map to symptoms and then create a conversational method for patients to report their symptoms in English. Here is my initial attempt to do so for one symptom. Look at how comprehensive the list produced by ChatGPT for the symptom “vomit”:
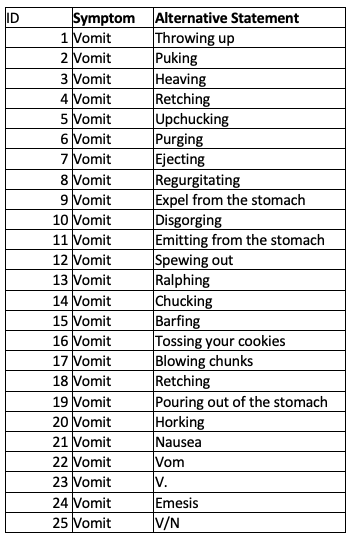
There are 25 different ways to say, “I vomited.” This does not include misspelled words and Spanish words. But that, too, is available through reverse engineering some parts of ChatGPT. The point is that the public version of ChatGPT provides the elements needed to create our own algorithm for understanding text and producing the recommendation.
We’re hearing a lot about ChatGPT for essay writing. Can Chat GPT be used for math and science?
We are working on a project to enable ChatGPT, or any large language model, to learn from statistics, in particular from regression models and analysis of variance. Some components of this are already available in ChatGPT, but not in sufficient detail. A lot of science is expressed in mathematics. Right now, this is beyond the public version of ChatGPT. So, as a consequence, the public version reports are not detailed and tailored enough to a patient’s needs. The public version does not learn from formulas, and teams of scientists are needed to build formulas and calculators into ChatGPT. When we do so, then the version of ChatGPT that is trained on PubMed can come closer to artificial intelligence helping with the diagnosis or treatment of patients.
To correctly answer patients’ questions, large language models need to be trained on formulas and on meta-analysis of statistical models, the fundamental method, and the language of science. Our work in this area is focused on translating our recent formula-based model for the diagnosis of COVID into ChatGPT. We are also working on creating a similar tool for the selection of antidepressants.