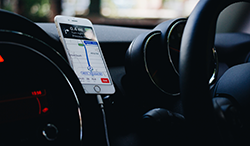
Study participants will use GPS apps to provide much-needed data on how people move during and after stay-at-home restrictions.
College of Health and Human Services launches study to model how the diseases spreads through each stage of the stay-at-home order.
For public health experts fighting COVID-19, there are still many unknowns including how the disease spreads through populations, what impact lifting the stay-at-home order may have on the infection rate, and how people’s movement patterns affect the spread of the disease.
Janusz Wojtusiak, associate professor of Health Informatics and director of the Machine Learning Inference Lab; Amira Roess, professor of Global Health and Epidemiology; and Dieter Pfoser, professor and chair of Geography and Geoinformation Science have launched a study to answer these questions. The goal of the study is to develop a better understanding of people’s movement patterns and ultimately model the potential spread of the pandemic.
There are two parts to the study. First, participants will use GPS apps to provide much-needed data on how people move during and after stay-at-home restrictions. Researchers will use this data to create models that predict movement at a larger scale and to help understand how people move under restrictions compared to when the pandemic is over. Second, study participants will use the Daily Symptoms Tracker throughout the study to record any symptoms of possible COVID-19 infection.
When combined, the dataset will provide researchers with a deeper understanding of movement patterns through various stages of the stay-at-home order and how those movements can spread the COVID-19 disease. Once this information is gathered at a small scale, experts in machine learning can develop models for how movement impacts the spread of the disease. Modeling different scenarios will be accomplished by preforming large-scale computer simulations guided by machine learning models created by study participants.
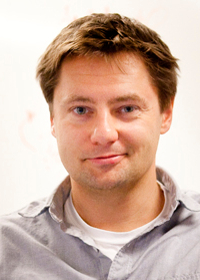
"Space-time (spatiotemporal) GPS data are complex and tell only part of the story about people’s movement. The data are further enriched by daily journal responses," says Wojtusiak.
Wojtusiak and collaborators will use the data gathered through the study to construct two types of models: 1) individual models that are tailored to each specific participant to better understand their specific movement patterns, typically related to weekly routines and visited locations; and 2) global models that can learn patterns across many people using aggregate data derived from maps. The latter will help us understand how much time people spend on activities such as doing groceries, walking around neighborhoods, and staying home.
“There are strong methodological and application components of the study,” explains Wojtusiak. “The project is driven by the immediate need to understand peoples’ movements during pandemics. But solving the study problems require new science in how data are analyzed to provide meaningful results. Space-time (spatiotemporal) GPS data are complex and tell only part of the story about people’s movement. The data are further enriched by daily journal responses. For example, we work on novel approaches to find out exactly how a person moves between GPS-recorded points on a map. We also expand capabilities of machine learning algorithms to build individual models that can predict movement and use these models to guide a large-scale computer simulation.”
Fighting COVID-19 requires a deep understanding of how the disease spreads through populations matched with the ability to proactively provide resources such as screening, contact tracing, and testing to stem outbreaks when infections are identified. This study seeks to provide public health officials with data to inform decisions about COVID-19 and possible future infectious disease outbreaks.
Study participants will receive data to help better understand their own movement patterns and will contribute to the larger understanding of movement patterns throughout the various stages of the stay-at-home order. For more information about the study, including confidentiality and privacy see the study overview and consent form. Results of the study will be disseminated in research reports and publications. At this moment the study is still open to new participants from Mason community. For more information, please view the consent form for the study.